New research optimizes energy density in sodium-ion batteries
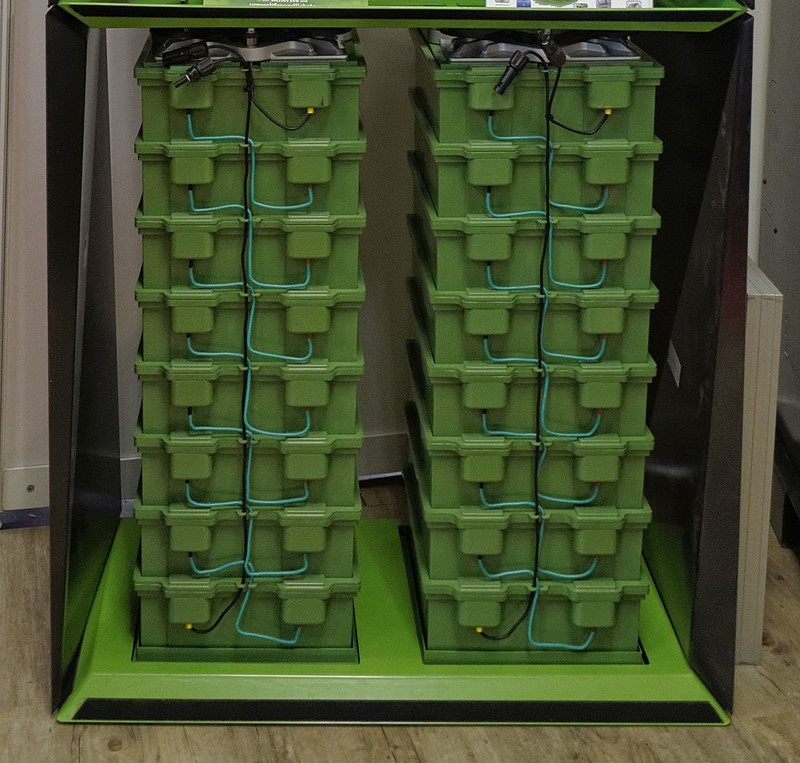
Scientists from Japan’s Tokyo University of Science (TUS) and Nagoya Institute of Technology, and from Chalmers University of Technology, in Gothenburg, Sweden, have developed a machine learning method to optimize the energy density of Na-ion batteries.
The research brings Na-ion batteries one step closer to becoming a commercially viable alternative to lithium-ion devices. Lithium-ion currently dominates the battery market but lithium’s relative scarcity, and high price, is a problem for supply chains and project developers alike.
Sodium’s abundance makes it a promising lower-cost – and potentially safer – alternative to lithium for battery use.
Sodium-containing transition-metal layered oxides (NaMeO2) are powerful materials for the positive electrodes of Na-ion batteries, which offer exceptional energy density and capacity. There is a caveat, however. For multi-element layered oxides composed of several transition metals, the number of possible combinations makes finding the optimal composition extremely difficult. Even minor changes in the selection and proportion of transition metals can bring about marked changes in crystal morphology and can affect battery performance.
The TUS-led researchers, however, claim to have identified Na[Mn0.36Ni0.44Ti0.15Fe0.05]O2 as the optimum composition for the highest energy density – one of the most important characteristics in electrode materials.
Model
The academics used a machine learning model to automate the complex screening process for elemental compositions of various NaMeO2 O3-type materials.
The researchers used the model on a database of 100 samples from O3-type sodium-half cells with 68 different compositions. These had been gathered over the course of 11 years by Professor Shinichi Komaba, of TUS, who co-led the study.
“The database included the composition of NaMeO2 samples – with Me [Messierium] being a transition metal like Mn [manganese], Ti [titanium], Zn [zinc], Ni [nickel] … Fe [iron], and Sn [tin], among others – as well as the upper and lower voltage limits of charge-discharge tests, initial discharge capacity, average discharge voltage, and capacity retention after 20 cycles,” said Komaba.

To verify the accuracy of their model’s prediction, Komaba and his team synthesized samples with Na[Mn0.36Ni0.44Ti0.15Fe0.05]O2 and assembled standard coin cells to run charge and discharge tests.
The researchers used the same database to train a model incorporating several machine learning algorithms as well as “Bayesian optimization,” a method which optimizes decision making, to perform an efficient search. The goal of this model was to learn how properties such as operating voltage, lifetime, and energy density are related to the composition of NaMeO2 layered oxides, and to predict the optimal ratio of elements needed to achieve a desired balance between these properties.
The measured values were mostly consistent with the predicted ones, proving the model’s potential as a method for exploring new battery materials. Komaba said, the approach “offers an efficient method to identify promising compositions from a wide range of potential candidates.”
“Moreover, this methodology is extendable to more complex material systems, such as quinary transition metal oxides,” he added.
The study has been published in the Journal of Materials Chemistry A.